题目来自老师的课后作业,如下所示。很多地方应该可以直接调用函数,但是初学Python,对里面的函数还不是很了解,顺便带着学习的态度,尽量自己动手code。
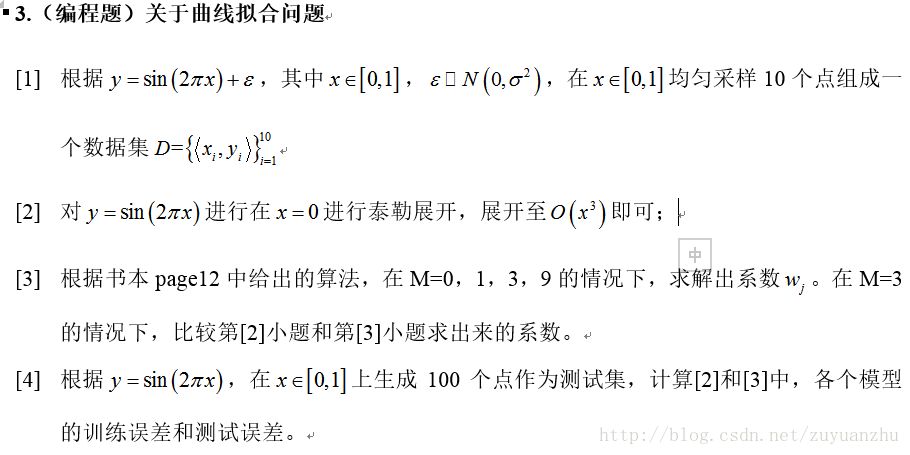
测试版代码,里面带有很多注释和测试代码:
- import math
- import random
- import matplotlib.pyplot as plt
- import numpy as np
-
-
- ''
- a = []
- b = []
- x=0
- def func(x):
- mu=0
- sigma=0.1
- epsilon = random.gauss(mu,sigma)
- return np.sin(2*np.pi*x)+epsilon
- for i in range(0,10):
- x=x+1.0/11.0
- a.append(x)
- b.append(func(x))
-
-
-
-
- def print_matrix( info, m ):
- i = 0; j = 0; l = len(m)
- print info
- for i in range( 0, len( m ) ):
- for j in range( 0, len( m[i] ) ):
- if( j == l ):
- print ' |',
- print '%6.4f' % m[i][j],
- print
- print
-
-
- def swap( a, b ):
- t = a; a = b; b = t
-
- def solve( ma, b, n ):
- global m; m = ma
- global s;
- i = 0; j = 0; row_pos = 0; col_pos = 0; ik = 0; jk = 0
- mik = 0.0; temp = 0.0
- n = len( m )
- while( ( row_pos < n ) and( col_pos < n ) ):
-
- mik = - 1
- for i in range( row_pos, n ):
- if( abs( m[i][col_pos] ) > mik ):
- mik = abs( m[i][col_pos] )
- ik = i
- if( mik == 0.0 ):
- col_pos = col_pos + 1
- continue
-
- if( ik != row_pos ):
- for j in range( col_pos, n ):
- swap( m[row_pos][j], m[ik][j] )
- swap( m[row_pos][n], m[ik][n] );
- try:
-
- m[row_pos][n] /= m[row_pos][col_pos]
- except ZeroDivisionError:
-
- return 0;
- j = n - 1
- while( j >= col_pos ):
- m[row_pos][j] /= m[row_pos][col_pos]
- j = j - 1
- for i in range( 0, n ):
- if( i == row_pos ):
- continue
- m[i][n] -= m[row_pos][n] * m[i][col_pos]
- j = n - 1
- while( j >= col_pos ):
- m[i][j] -= m[row_pos][j] * m[i][col_pos]
- j = j - 1
- row_pos = row_pos + 1; col_pos = col_pos + 1
- for i in range( row_pos, n ):
- if( abs( m[i][n] ) == 0.0 ):
- return 0
- return 1
-
-
-
-
- matrix_A=[]
- matrix_b=[]
- X=a
- Y=b
- N=len(X)
- M=3
-
-
- def matrix_element_A(x,i,j,n):
- sum_a=0
- for k in range(0,n):
- sum_a = sum_a+pow(x[k],i+j-2)
- return sum_a
-
-
- for i in range(0,M+1):
- matrix_A.append([])
- for j in range(0,M+1):
- matrix_A[i].append(0)
- matrix_A[i][j] = matrix_element_A(X,i+1,j+1,N)
- def matrix_element_b(x,y,i,n):
- sum_b=0
- for k in range(0,n):
- sum_b=sum_b+y[k]*pow(x[k],i-1)
- return sum_b
- for i in range(0,M+1):
- matrix_b.append(matrix_element_b(X,Y,i+1,N))
-
-
- def matrix_element_A_(array_A,array_b,A_row):
- M=A_row
- matrix_A_= []
- for i in range(0,M+1):
- matrix_A_.append([])
- for j in range(0,M+2):
- matrix_A_[i].append(0)
- if j<M+1:
- matrix_A_[i][j] = array_A[i][j]
- elif j==M+1:
- matrix_A_[i][j] = array_b[i]
- return matrix_A_
- matrix_A_ = matrix_element_A_(matrix_A,matrix_b,M)
-
-
- ''
- def poly_fit(x,wp,m):
- sumf = 0
- for j in range(0,m+1):
- sumf=sumf+wp[j]*pow(x,j)
- return sumf
-
-
- ''
- coef_taylor = []
- K=3
- if K%2==0:
- print "K必须为正奇数"
- s = 0
- k=(K-1)/2+1
- for i in range(0,k):
- s = pow(-1,i)*pow(2*np.pi,2*i+1)/math.factorial(2*i+1)
- coef_taylor.append(s)
- print "%d阶泰勒级数展开式的系数为:" %K
- print coef_taylor
- def sin_taylor(tx):
- sum_tay=0
- for i in range(0,k):
- sum_tay=sum_tay+coef_taylor[i]*pow(tx,2*k+1)
- return sum_tay
- poly_taylor_a = []
- poly_taylor_b = []
- for i in range(0,N):
- poly_taylor_a.append(a[i])
- poly_taylor_b.append(sin_taylor(poly_taylor_a[i]))
-
-
-
-
- ''
- testa = []
- testb = []
- x=0
- for i in range(0,100):
- x=x+1.0/101.0
- testa.append(x)
- testb.append(np.sin(2*np.pi*x))
-
- ''
- def Lfun(ly,fx):
- L=0
- for i in range(0,len(fx)):
- L=L+pow(ly[i]-fx[i],2)
- return L
-
-
- ''
- if __name__ == '__main__':
- ret = solve( matrix_A_, 0, 0 )
- if( ret== 0 ):
- print "方 程组无唯一解或无解\n"
-
-
- w = []
- for i in range( 0, len( m ) ):
- w.append(m[i][len( m )])
- print "M=%d时的系数w[j]:" %M
- print w
-
-
- poly_a = []
- poly_b = []
- for i in range(0,N):
- poly_a.append(a[i])
- poly_b.append(poly_fit(poly_a[i],w,M))
-
-
-
- fxtay = []
- for i in range(0,100):
- fxtay.append(sin_taylor(testa[i]))
- LCtaylor = Lfun(testb,fxtay)/100
- print "三阶泰勒展开式的测试误差为:%f" %LCtaylor
-
-
-
- fxpoly = []
- for i in range(0,N):
- fxpoly.append(poly_fit(a[i],w,M))
- LXpoly = Lfun(b,fxpoly)/len(poly_b)
- print "M=%d时多项式拟合函数的训练误差为:%f" % (M,LXpoly)
-
-
-
- fxpolyc = []
- for i in range(0,100):
- fxpolyc.append(poly_fit(testa[i],w,M))
- LCpoly = Lfun(testb,fxpolyc)/100
- print "M=%d时多项式拟合函数的测试误差为:%f" % (M,LCpoly)
-
-
- fig1 = plt.figure(1)
- plt.plot(poly_a,poly_b,color='blue',linestyle='solid',marker='o')
-
- plt.plot(a,b,color='red',linestyle='dashed',marker='x')
-
- plt.plot(poly_taylor_a,poly_taylor_b,color='yellow',linestyle='dashed',marker='o')
-
- fig2 = plt.figure(2)
- plt.plot(poly_a,poly_b,color='blue',linestyle='solid',marker='o')
- plt.plot(a,b,color='red',linestyle='dashed',marker='x')
- plt.show()
M=3时的运行结果:
- 3阶泰勒级数展开式的系数为:
- [6.283185307179586, -41.341702240399755]
- M=3时的系数w[j]:
- [-0.28492708632293295, 13.031310645420685, -37.730992850050448, 25.464782221275197]
- 三阶泰勒展开式的测试误差为:100.889335
- M=3时多项式拟合函数的训练误差为:0.008933
- M=3时多项式拟合函数的测试误差为:0.007886
Figure(1):
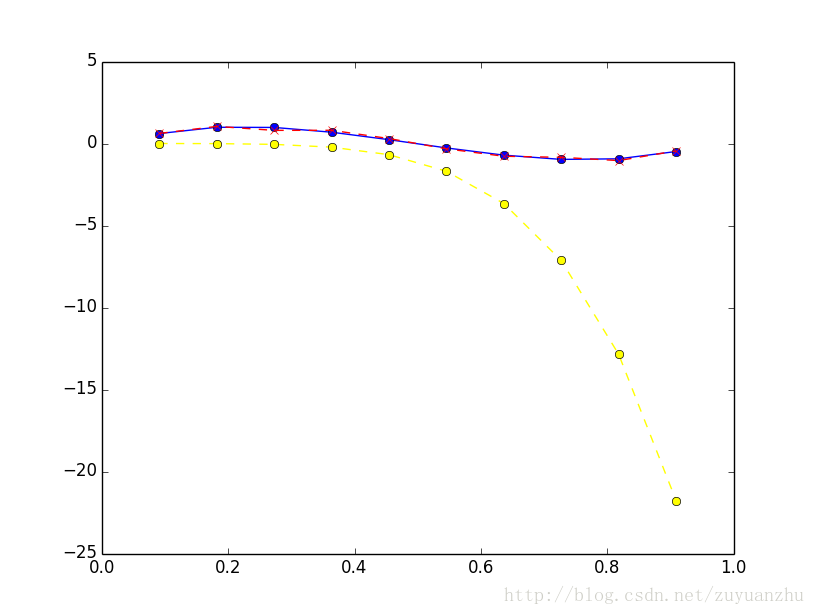
Figure(2):
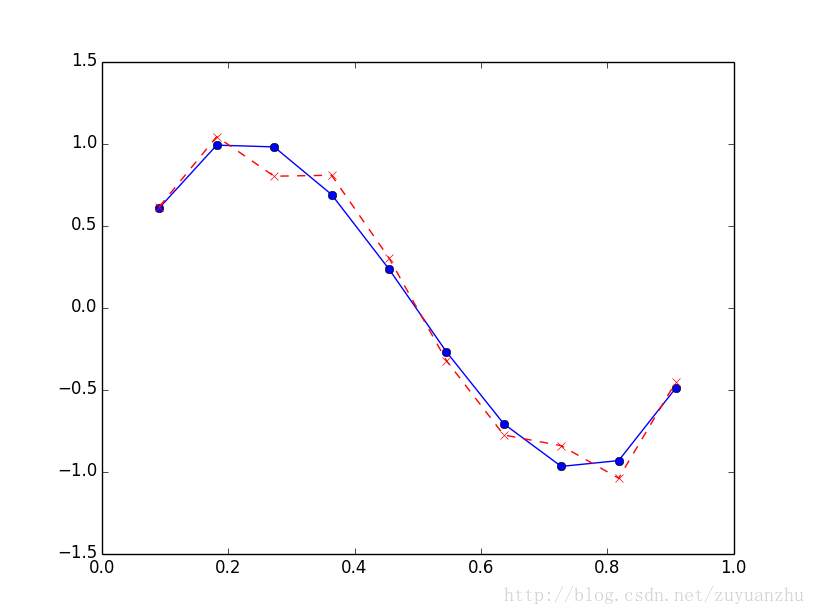
初次编写这么长的代码,思路不是有一点的混乱
。其中有
也有
。以后会继续来优化这个程序,作为学习Python的入口。
http://blog.csdn.net/zuyuanzhu/article/details/21321007